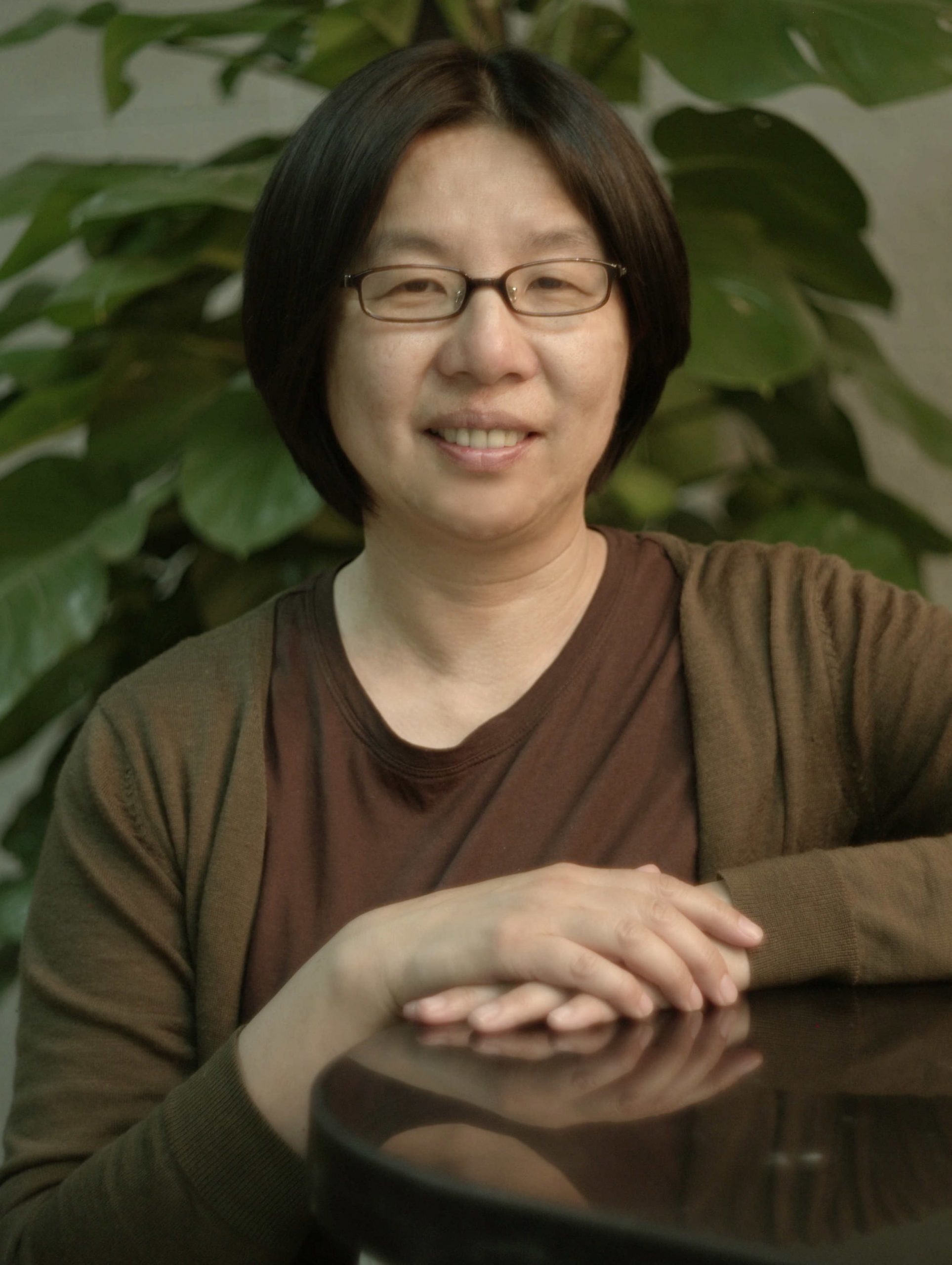
Speaker: Bin Yu
Affiliation: UC Berkeley
ABSTRACT:
Veridical data science extracts reliable and reproducible information from data, with an enriched technical language to communicate and evaluate empirical evidence in the context of human decisions and domain knowledge. Building and expanding on principles of statistics, machine learning, and the sciences, we propose the predictability, computability, and stability (PCS) framework for veridical data science. Our framework is comprised of both a workflow and documentation and aims to provide responsible, reliable, reproducible, and transparent results across the entire data science life cycle.
Moreover, we propose the PDR desiderata for interpretable machine learning as part of veridical data science (with PDR standing for predictive accuracy, predictive accuracy and relevancy to a human audience and a particular domain problem).
The PCS framework will be illustrated through the development of the DeepTune framework for characterizing V4 neurons. DeepTune builds predictive models using DNNs and ridge regression and applies the stability principle to obtain stable interpretations of 18 predictive models. Finally, a general DNN interpretaion method based on contextual decomposition (CD) will be discussed with applications to sentiment analysis and cosmological parameter estimation.
BIO:
Bin Yu is Chancellor’s Professor in the Departments of Statistics and of Electrical Engineering & Computer Sciences at the University of California at Berkeley and a former chair of Statistics at UC Berkeley. Her research focuses on practice, algorithm, and theory of statistical machine learning and causal inference. Her group is engaged in interdisciplinary research with scientists from genomics, neuroscience, and precision medicine. In order to augment empirical evidence for decision-making, she and her group are investigating methods/algorithms (and associated statistical inference problems) such as dictionary learning, non-negative matrix factorization (NMF), EM and deep learning (CNNs and LSTMs), and heterogeneous effect estimation in randomized experiments (X-learner). Their recent algorithms include staNMF for unsupervised learning, iterative Random Forests (iRF) and signed iRF (s-iRF) for discovering predictive and stable high-order interactions in supervised learning, contextual decomposition (CD) and aggregated contextual decomposition (ACD) for phrase or patch importance extraction from an LSTM or a CNN.
She is a member of the U.S. National Academy of Sciences and Fellow of the American Academy of Arts and Sciences. She was a Guggenheim Fellow in 2006, and the Tukey Memorial Lecturer of the Bernoulli Society in 2012. She was President of IMS (Institute of Mathematical Statistics) in 2013-2014 and the Rietz Lecturer of IMS in 2016. She received the E. L. Scott Award from COPSS (Committee of Presidents of Statistical Societies) in 2018. She was a founding co-director of the Microsoft Research Asia (MSR) Lab at Peking University and is a member of the scientific advisory board at the Alan Turning Institute in the UK.
Hosted By: Tao Gao (Statistics) and Quanquan Gu (Computer Science)
Date/Time:
Date(s) - Jan 14, 2020
11:00 am - 12:00 pm
Location:
Mong Auditorium – Engineering VI – First Floor
404 Westwood Blvd Los Angeles California 90095