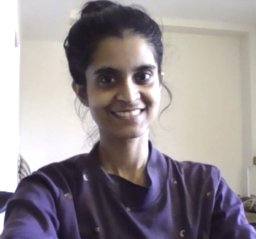
Speaker: Aadirupa Saha
Affiliation: Apple ML Research
ABSTRACT:
In this talk we will discuss an unconventional version of the standard bandit convex optimization (BCO) problem with preference (dueling) feedback—Like the traditional optimization objective, the goal is to find the minimizer of a convex function with the least possible query complexity, however, without the luxury of even zeroth-order feedback; here instead, at each round, the learner can only observe a single noisy 0/1 win-loss feedback for a pair of queried points (based on their underlying function values). The problem is certainly of great practical relevance in any real-world system with potentially large (or even infinite) decision spaces.
The main difficulty in this framework is that any “gradient-descent” based technique is bound to fail as it is impossible to estimate gradient information at any point from a single-bit comparison preference feedback which does not reveal any magnitude information of the underlying cost function at the queried points. We overcome this challenge by designing normalized gradient descent-based algorithms and showed near-optimal convergence rates for smooth and strongly convex functions. Towards the end, we will consider this problem for a very general class of preference functions which includes all monotonic functions that can be approximated by finite degree polynomials. We will conclude the talk with a plethora of open questions led by this general direction of optimization with `unconventional feedback’ which can help bridging the gaps between theory and practice in many real-world applications.
[Based on joint works with Tomer Koren (Tel Aviv Univ and Google), Yishay Mansour (Tel Aviv Univ and Google)]
BIO:
Aadirupa is currently a research scientist at Apple ML research, broadly working in the area of Machine Learning theory. She just finished a short-term research visit at Toyota Technological Institute at Chicago (TTIC), and completed her postdoc stinct at Microsoft Research New York City before that. Aadirupa obtained her P.h.D from the department of Computer Science, Indian Institute of Science, Bangalore, advised by Aditya Gopalan and Chiranjib Bhattacharyya and interned at Microsoft Research, INRIA Paris, and Google AI.Her research interests include Bandits, Reinforcement Learning, Optimization, Learning theory, Algorithms. Off late, she is also very interested in working on problems at the intersection of ML and Game theory, Algorithmic fairness, and Privacy.
Hosted by Professor Quanquan Gu
Date/Time:
Date(s) - Jun 01, 2023
4:15 pm - 5:30 pm
Location:
3400 Boelter Hall
420 Westwood Plaza Los Angeles California 90095