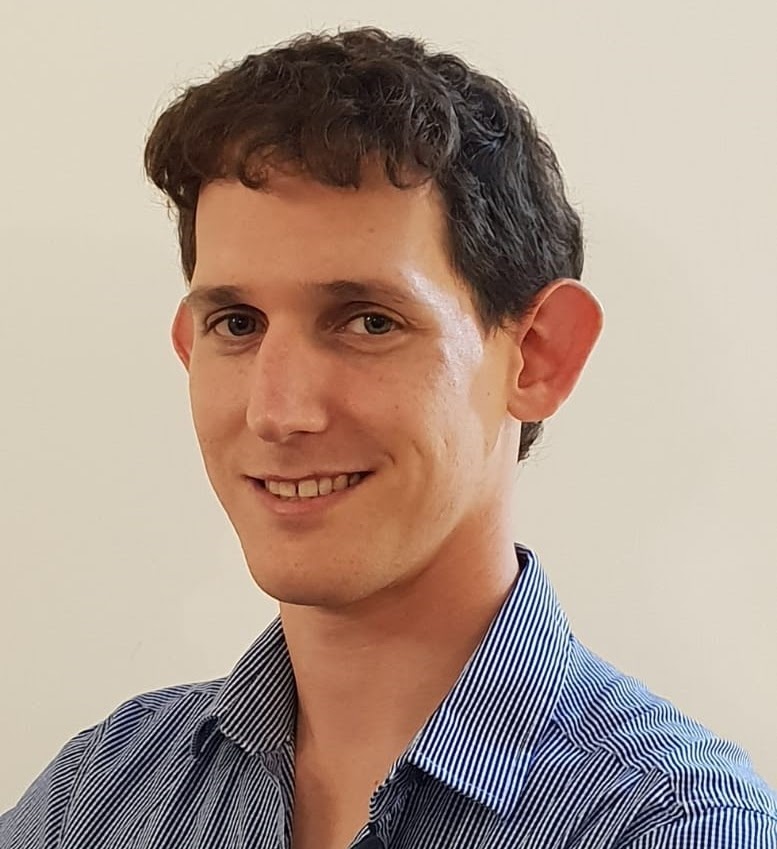
Speaker: Gal Vardi
Affiliation: Toyota Technological Institute of Chicago
ABSTRACT:
When training large neural networks, there are generally many weight combinations that perfectly fit the training data. However, gradient-based training methods somehow tend to reach those which generalize well, and understanding this “implicit bias” has been a subject of extensive research. In this talk, I will discuss three recent works which show settings where the implicit bias provably implies generalization (in two-layer neural networks trained with gradient flow w.r.t. the logistic loss): First, the implicit bias implies generalization in univariate ReLU networks. Second, in ReLU networks where the data consists of clusters and the correlations between cluster means are small, the implicit bias leads to solutions that generalize well, but are highly vulnerable to adversarial examples. Third, in Leaky-ReLU networks (as well as linear classifiers), under certain assumptions on the input distribution, the implicit bias implies benign-overfitting: the estimators interpolate noisy training data and simultaneously generalize well to test data.
Based on joint works with Spencer Frei, Itay Safran, Peter L. Bartlett, Jason D. Lee, and Nati Srebro.
BIO:
Gal is a postdoc at TTI-Chicago and the Hebrew University, hosted by Nati Srebro and Amit Daniely as part of the NSF/Simons Collaboration on the Theoretical Foundations of Deep Learning. Prior to that, he was a postdoc at the Weizmann Institute, hosted by Ohad Shamir, and a PhD student at the Hebrew University advised by Orna Kupferman. His research focuses on theoretical machine learning, with an emphasis on deep-learning theory.
Hosted by Professor Quanquan Gu
Date/Time:
Date(s) - Mar 14, 2023
4:00 pm - 5:45 pm
Location:
Zoom Webinar
404 Westwood Plaza Los Angeles
Map Unavailable