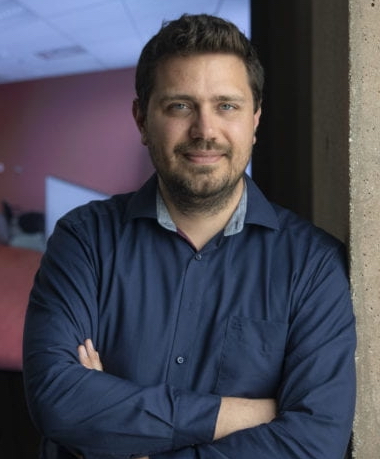
Speaker: Stephan Mandt
Affiliation: UC Irvine
ABTRACT:
Latent variable models have been an integral part of probabilistic machine learning, ranging from simple mixture models to variational autoencoders to powerful diffusion probabilistic models at the center of recent media attention. Perhaps less well-appreciated is the intimate connection between latent variable models and compression, and the potential of these models for advancing natural science. This talk will explore these topics. I will begin by showcasing connections between variational methods and the theory and practice of neural data compression. On the applied side, variational methods lead to machine-learned compressors of data such as images and videos and offer principled techniques for enhancing their compression performance, as well as reducing their decoding complexity. On the theory side, variational methods also provide scalable bounds on the fundamental compressibility of real-world data, such as images or particle physics data. Finally, I will describe how latent variable concepts can advance natural science, from chemistry to climate modeling.
BIO:
Stephan Mandt is an Associate Professor of Computer Science and Statistics at the University of California, Irvine. From 2016 until 2018, he was a Senior Researcher and Head of the statistical machine learning group at Disney Research in Pittsburgh and Los Angeles. He held previous postdoctoral positions at Columbia University and Princeton University. Stephan holds a Ph.D. in Theoretical Physics from the University of Cologne, where he received the German National Merit Scholarship. He is furthermore a recipient of the NSF CAREER Award, the UCI ICS Mid-Career Excellence in Research Award, the German Research Foundation’s Mercator Fellowship, a Kavli Fellow of the U.S. National Academy of Sciences, a member of the ELLIS Society, and a former visiting researcher at Google Brain. His research is currently supported by NSF, DARPA, IARPA, DOE, Disney, Intel, and Qualcomm. Stephan is an Action Editor of the Journal of Machine Learning Research and Transaction on Machine Learning Research and regularly serves as an Area Chair for NeurIPS, ICML, AAAI, and ICLR. He currently serves as Program Chair for AISTATS 2024 and will continue to serve AISTATS as General Chair in 2025.
Hosted by Professor Aditya Grover
Date/Time:
Date(s) - Oct 12, 2023
4:15 pm - 5:45 pm
Location:
3400 Boelter Hall
420 Westwood Plaza Los Angeles California 90095